Many companies use the data they have to get insights, for example on how to grow their business. Unfortunately, a lack of data variety and superficiality in the analysis can often lead to the wrong conclusion.
One quick and simple example can be produced by creating 100 imaginary voters and assigning votes to them.
Let’s say we have 50 men and 50 women, of which we have 40 white and 10 black. Let us assume that in the white population, both men and women, 25 out of 40 people vote, and of these, 15 vote “R” and 10 vote “D”. Let’s also assume that we have only 1 out of 10 black men voting, and voting “D”, and 9 out of 10 black women voting, and all also voting “D”.
So we have:
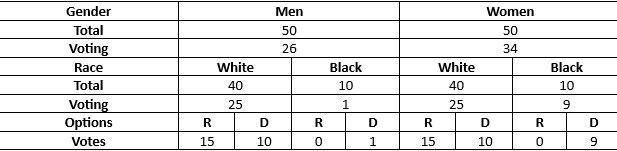
We can aggregate the data by gender and we get:

This clearly shows that, though “R” and “D” get 50% of the votes, more women vote “D” and more men vote “R”. One could then draw the conclusion that, in order for “D” to win, they need to push more women to vote because they lean towards “D”.
This is clearly a mistake that can be easily made, and it is caused by the lack of understanding the data and lack of variety in the way it was aggregated.
White women vote 60% for “R” and only 40% for “D” in the data. It is true that women overall lean towards “D”, however this is because of black women who, however, already reach a 90% participation (9 out 10 vote) and are unlikely to grow much more. Pushing women to vote would, most likely, push more white women to vote, probably favouring “R” over “D”, the opposite of the stated goal.
In fact, a better understanding of the data shows that the real discriminant between “R” and “D” is not gender, rather it is race.
If we aggregate the data by race, we see:

In addition, black men participation is only 10%, therefore it could grow more, and they also vote predominantly “D”. A better strategy would then be to push men to vote more, and in particular more black men.
If “D” and “R” were competing priorities for a company, choosing the wrong strategy could actually bring diminished results and revenues.
As I mentioned in my previous post on “How to handle big data”, variety is often necessary to get better insights, as this example shows, whereas a lack of variety and, in particular, race information, could suggest an incorrect strategy.